Kea are known to be smart and the more we learn about them, the smarter we realise they are. But did you know kea can do maths?
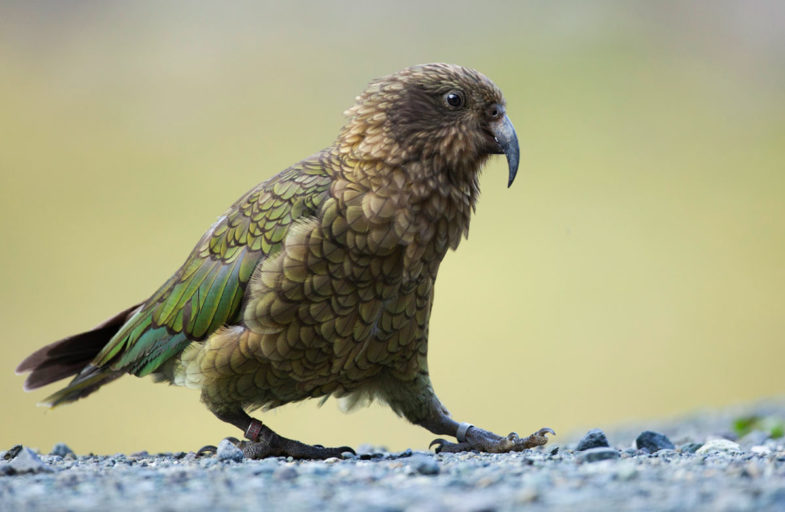
Yes – it turns out that kea are pretty good at basic mathematical statistics – especially when it comes to calculating the odds of getting the reward they want. They can even recognise a sampling bias in their human experimenters and go with the human who is more likely to be hiding a treat token in their hand.
Amalia Bastos and Alex Taylor from Auckland University’s School of Psychology devised a series of activities to put kea cognition to the test and found out they were very sophisticated thinkers – on par with apes and human infants. The study was based on the domain-general learning theories of development which (according to Wikipedia) suggest that humans are born with mechanisms in the brain that exist to support and guide learning on a broad level, regardless of the type of information being learned.
And not just humans it seems…
“One key aspect of domain-general thought is the ability to integrate information across different cognitive domains. Here, we tested whether kea (Nestor notabilis) can use relative quantities when predicting sampling outcomes, and then integrate both physical information about the presence of a barrier, and social information about the biased sampling of an experimenter, into their predictions.”
The researchers used two types of tokens in their stats test – rewarding tokens and unrewarding tokens. In the first series of experiments, the tokens were mixed together in jars. Some jars had a greater proportion of rewarding tokens than others – meaning that a random sample taken from those jars was more likely to be a rewarding token than not. So can kea calculate probability?
You bet they can!
“Over the course of three conditions, we tested whether kea could make sampling predictions using relative rather than absolute quantities. In Condition 1, subjects were presented with two populations, one with 100 rewarding tokens and 20 unrewarding tokens, the other with these frequencies reversed. Kea observed an experimenter sampling from each of these populations and were then presented with two closed hands. Three of six kea spontaneously showed a preference for the hand that had sampled the population with 100 rewarding tokens within their first 20 trials.”
Like humans, some kea seem to catch on to maths more quickly than others. But eventually they all got it.
“We then gave all kea experience with this task until they chose the hand sampling the population with 100 rewarding tokens in 17/20 trials, which took kea 120 trials on average. Condition 2 tested if kea were using an absolute quantity-based heuristic by selecting the jar with the most rewarding tokens. Here, kea had to choose between 2 hands that both sampled from a population containing 20 rewarding tokens. However, one population had 100 unrewarding tokens, and the other had 4 unrewarding tokens. Four kea chose the jar with fewer unrewarding tokens within their first 20 trials. Again, we gave subjects experience with this task until they reached a 17/20 criterion, which took 66.67 trials on average.”
The calculation of ‘best odds’ of getting a rewarding token got increasingly sophisticated as the experiments continued.
“Condition 3 controlled for a second absolute quantity-based heuristic, the avoidance of the jar with the most unrewarding tokens, a control which capuchins fail. Kea observed sampling from one jar which contained 63 unrewarding tokens and 57 rewarding tokens and a second that again contained 63 unrewarding tokens but only 3 rewarding tokens. All 6 kea chose the jar with 57 rewarding tokens above chance within their first 20 trials.”
Smarter than capuchin monkeys then – and this time all six kea quickly worked out the best odds. Together these three conditions made up the first experiment and showed that kea can do stats.
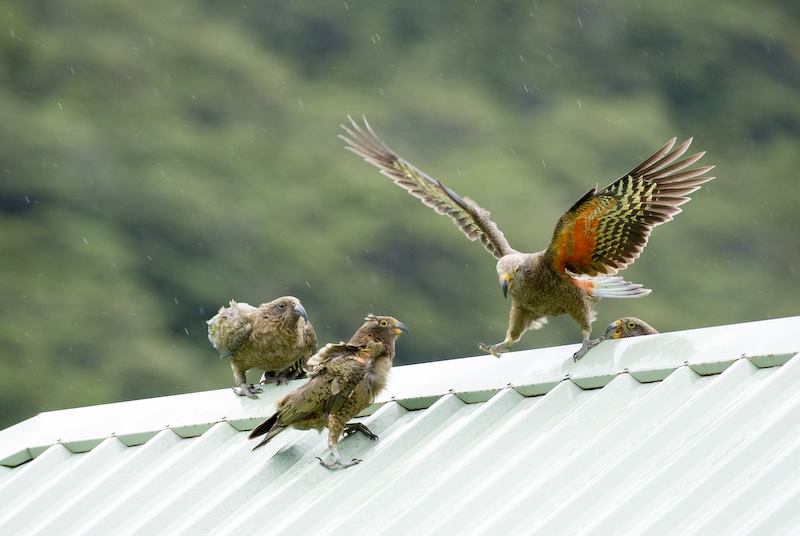
“The results of Experiment 1 provide conclusive evidence that kea show true statistical inference using the relative frequency of items, rather than using quantity heuristics based on the absolute number of items. Four of our six subjects performed above chance within their first 20 trials of both Conditions 2 and 3, indicating they had not learnt during their past experience to use a heuristic based on choosing the population with either the most rewarding, or most unrewarding items. Kea therefore did not behave as capuchins do, in using the absolute number of positive or negative tokens within the jars to make decisions. Instead, kea mirrored the performance of infants and chimpanzees in using the relative frequency of objects across this experiment.”
Time to up the ante…
“Experiment 2 tested whether kea could integrate information about a physical constraint into their prediction of a sampling event, as infants do. After giving kea experience of a barrier, we presented them with two tasks where two jars, each with a barrier placed half-way down the jar, were sampled from. Each jar contained identical overall populations of tokens (80 tokens total: 40 rewarding and 40 unrewarding) but the proportions differed above and below the barriers.”
The barrier meant that the human experimenter could only choose a token from the upper portion of the jar above the barrier – so would kea make their choice based on what was in the whole jar or would they factor the barrier into their calculations? These birds are smart and it turned out that barriers are no barrier to them. Again they soon learnt to factor the barrier into their calculation of odds.
“Experiment 2 tested if kea could simultaneously integrate information about relative frequency with the presence of a barrier in order to make a judgement, because relying solely on one source of information—either the presence of a barrier, or the overall frequency of the population—would have led to the incorrect choice. These results not only confirm the results of Experiment 1, in showing kea use the relative frequency of objects to make statistical inference, but also show that kea can flexibly integrate physical knowledge into these inferences. When a barrier was placed in the jar, kea used only the relative frequency above the barrier when deciding which hand was more likely to contain a rewarding token.”
Finally, in Experiment 3, the researchers added some human bias. Are kea smart enough to assess human behavioural bias and factor that in too?
“Experiment 3 investigated whether kea could integrate social information about sampler biases into their predictions. We closely matched the procedure used in chimpanzees, providing kea with experience of a biased and an unbiased sampler. We first tested whether kea could distinguish between two human experimenters. Kea observed one experimenter closing their hand over a token and another experimenter who held nothing in their closed fist. The experimenters then either switched position or stayed in the same place and the kea was given the choice of one of the two experimenters’ hands. All kea passed this task above chance within 1 session of 20 trials.”
The next part of the experiment ensured that kea didn’t have any bias of their own towards preferring one experimenter over the other.
“We then tested kea for pre-existing preferences for one of the two experimenters in a token-exchange task, where both experimenters requested a rewarding token for exchange simultaneously and kea could select which of the two experimenters to obtain a reward from. We continued with this training until kea selected one of the experiments at between 9/20 and 11/20, which they all did within three sessions.”
Next, the kea were introduced to ‘experimenter bias’ among their humans. This bit gets more complicated even for a human brain.
“While the biased sampler selected rewarding tokens from a population of 110 tokens containing 10 rewarding and 100 unrewarding tokens, the unbiased sampler selected rewarding tokens from a population of 10 unrewarding and 100 rewarding tokens. Therefore, during demonstrations, both samplers were equally associated with a rewarding sampling outcome: both experimenters always sampled a rewarding token, but whilst the biased sampler did so by looking into a population with a minority of rewarding tokens, the unbiased sampler did so by blindly sampling from a population with a majority of rewarding tokens.”
So one (biased) human is coming up with the reward tokens by carefully choosing them against the odds. The other human is choosing randomly, but getting the reward tokens because the odds are in their favour. Can the kea suss what’s happening here and apply that learning when both their humans are given new jars of equal-mix tokens?
“At test, kea observed as the same two samplers picked from populations with an equal number of rewarding and unrewarding tokens (55 rewarding and 55 unrewarding tokens). If kea continued to use the relative frequencies of the tokens in each jar, we expected them to choose at chance. In contrast, if the kea understood that the biased sampler was indeed biased to choose a rewarding token, while the unbiased sampler had only been choosing rewarding tokens at the same frequency as the biased sampler due to the populations they were sampling from, kea should choose the biased sampler at test. This was because while the unbiased sampler would now be likely to choose a rewarding token half the time, the biased sampler should continue to choose the rewarding token in every trial.”
And yes – they did it. Kea worked out which human was choosing deliberately and therefore most likely to deliver the rewards.
“In order to succeed at this task, kea would need to integrate the social knowledge acquired during the demonstration phase into their probabilistic sampling predictions. Three of the six kea chose the biased sampler above chance at test. None of these three kea had previously shown a preference for either experimenter in the token-exchange task or during the demonstration phase. These results therefore mirror those from infants and chimpanzees in showing that kea can integrate social information on sampler biases into their statistical inferences.”
So when it comes to cognition – our bird-brain kea are up there with human infants and apes.
“Just like infants and the great apes, kea made statistical inferences using relative rather than absolute quantities, and then integrated social and physical information into their predictions, using their knowledge of physical barriers and the bias of a sampler, to override predictions based purely on relative probabilities.”
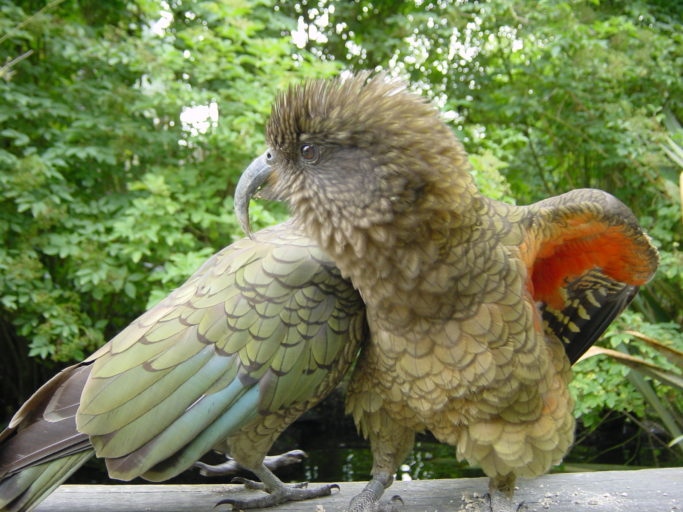
Studies like this not only show what a truly remarkable bird the kea is. They also throw light onto how intelligence may have evolved.
“Birds last shared a common ancestor with humans at least 312 million years ago. This evolutionary distance suggests that domain-general statistical inference may have arisen twice on our planet via convergent evolution. However, further work is required over a wider range of avian and primate species to provide a more accurate evolutionary account of when statistical inference has emerged in different taxa, and so test this hypothesis further.”
Bird brains are known to be structurally quite different from mammal brains.
“The statistical inference abilities observed in our study are particularly interesting given that birds have brains with a much smaller absolute size than humans, a very different structure, and much higher neuronal densities. Our results therefore suggest that (i) aspects of domain general thought can evolve multiple times, rather than being a one off or a product of a highly unlikely sequence of evolutionary events and (ii) that a particular brain architecture, specifically a layered cortex, is not necessary for this type of higher-order intelligence to evolve. This has important implications not only for our understanding of how intelligence evolves, but also for research focused on creating artificial, domain general thought processes (artificial general intelligence), specifically on the degree to which such processes should mirror mammalian cortical processes and structures.”
Artificial intelligence based on kea brain structure – one day, who knows… One thing is certain – our kea is one very special bird.
The full research article is Open Access, published online in Nature Communications.
Kea show three signatures of domain-general statistical inference (2020)